Quality control. Supply chain and logistics. Historical data. All these aspects have always had a strong role in the manufacturing industry, but new factors are coming into play to make manufacturing more efficient and low waste than ever before. Using the crossroads of the Internet of Things or Industrial IoT, artificial intelligence and predictive analytics, machine learning is the process of using deep learning in a smart manufacturing process to handle predictive maintenance, reducing equipment failure, ensure superior product quality, better manage industrial robots, increase machine intelligence, lower maintenance costs and keep up with consumer demand. But what is machine learning, exactly, and how will it impact your production line in the not-so-distant future?
How Will Machine Learning in Manufacturing Impact the Manufacturing Sector?
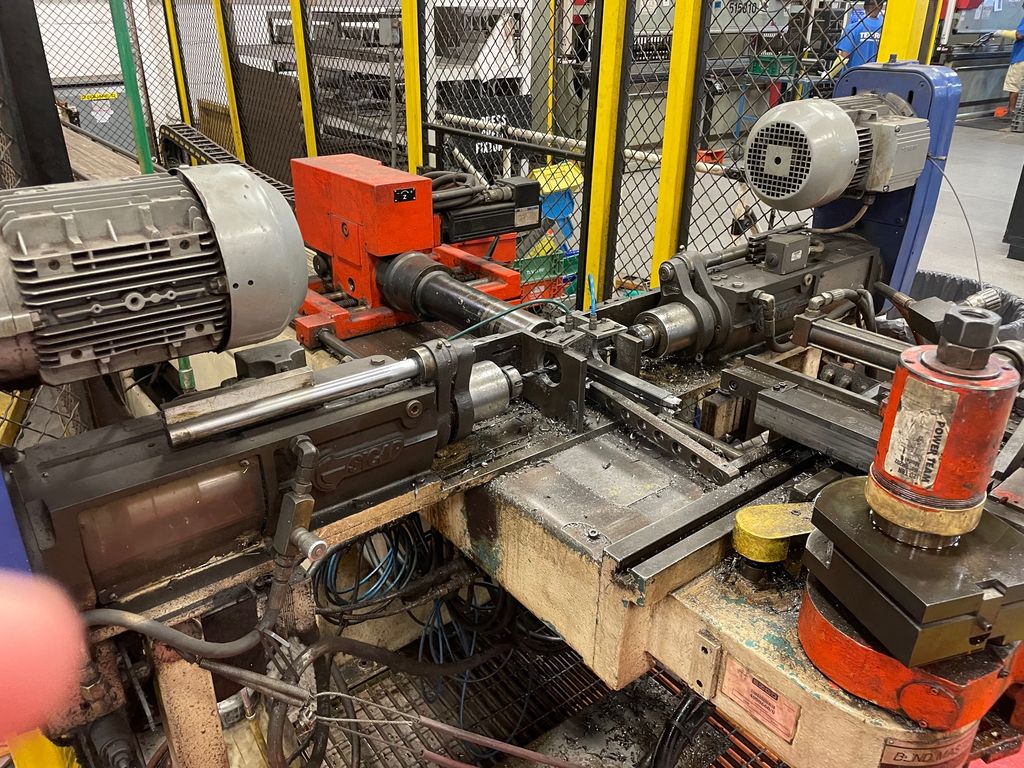
Let’s start by looking at the industrial revolution. In actuality, there have been four: the first, adding mechanization to industry, the second, which added electric power, the third, which added computing power, and the fourth, which adds pervasive networking to combine additive manufacturing, big data’s advanced analytics, real time industrial data, the Internet of Things which connects machine sensors to an analytic, electronic, artificial neural network, machine learning algorithms, cloud computing, and much more to improve predictive insights, remove labor and improve competitive advantage for companies that effectively embrace digital transformation.
Prior to digital transformation, also known as Industry 4.0 or Industrial Revolution 4.0, the manufacturing sector had a production process that went something like this:
- Industrial operations would start on an assembly line where somebody has an idea and thought they’d worked out the major issues.
- Supply chain management would be used to bring in raw materials needed to create the product. Sometimes it would take a great deal of time and effort during a shortage, and sometimes the materials received would not be up to spec.
- Somewhere along the line, quality issues would show up, and the industrial manufacturing process would adopt quality control options that would improve the quality of the finished product.
- New experimental results would provide additional adaptations to the product, resulting in new features.
- As the product became more mainstream, energy consumption would rise as the assembly line was kept open longer while labor costs increased to keep up with demand.
- As machinery broke down, the entire line would screech to a stop, with labor costs increasing against productivity.
But how do we change to the new opportunities that abound under digital transformation? Let’s take a minute to see what a post-transformation production process may look like:
- Once a basic idea has been created, it’s fed into a computer model to develop predictive models that take stress, usage, environmental and other factors that can cause the concept to fail. The design process isn’t complete until there is a solid product that will already pass most quality control processes.
- An automated supply chain process ensures that there is a consistent supply of raw materials to create the new product. Monitored inventory levels leads to automatic reordering of tested materials prior to running out, with poor-quality shipments automatically rejected before hitting the assembly line.
- An automated assembly line with industrial robots and an artificial neural network not only uses machine learning techniques and reinforcement learning to improve industrial applications, but also uses data analytics to improve energy efficiency, scale production to meet customer demand, provide messaging for anomaly detection, schedule automatic maintenance and predicts downtime issues approaching.
- Continuous feedback from social media, online reviews, automated testing and similar approaches allow for the release of new versions of the product with additional features, designs and similar aspects influenced by the online community that is using the product in the real world.
The Advantages of Machine Learning in Manufacturing
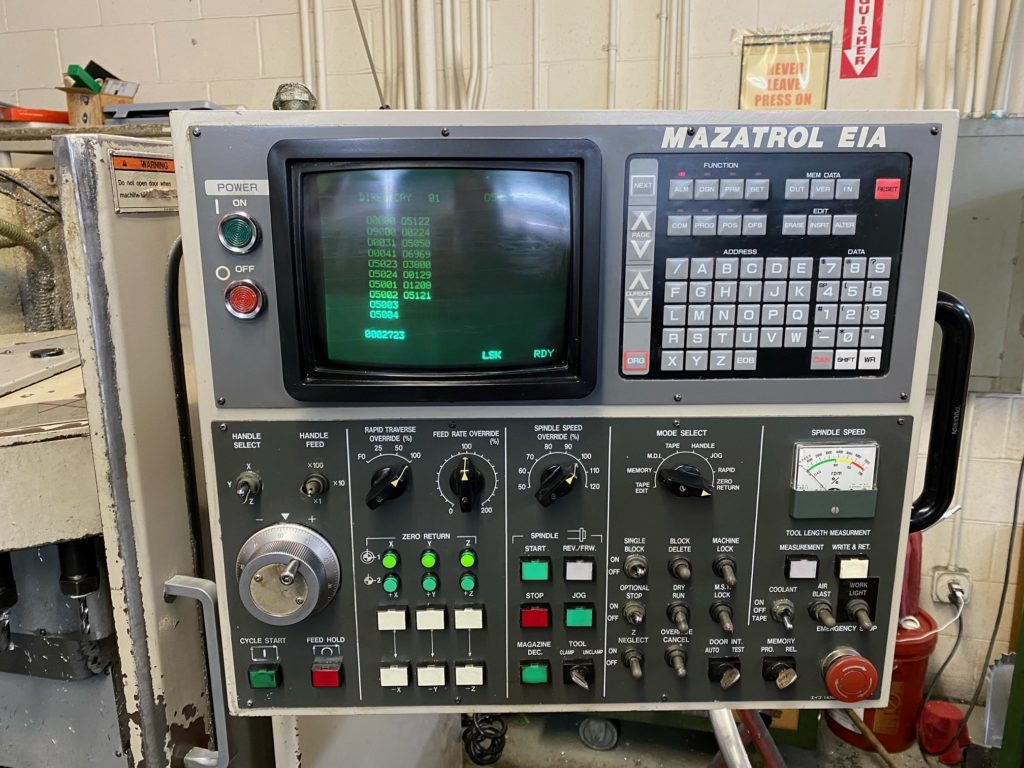
1. Lower Labor Costs
When manufacturing plants have been operated in the past, it’s typically included a very large labor expense for the business, as people are needed to operate the machines that assemble the products. Human eyes had to be able to observe when a welding robot was starting to put up too much splatter which would result in increased cleanup further down the line. They had to hit the button to stop the robot when it suddenly had an error code or to change its programming out because the line was moving from two-door to four-door models for a few days. They had to make sure that the machines were doing what they were supposed to, preferably without introducing human error into the mix. Modern machine learning allows the machines to oversee themselves, only reporting when there is a problem that requires a human operator to fix.
This drastically lowers the amount of labor needed to keep the plant running, not only on the assembly line, but also in other areas of the business. For example, the industrial robots supplying the line can keep track of when it’s getting lower on critical parts or raw materials, which are then automatically ordered also using the networked systems. The purchase order is automatically routed to accounts payable, which may have additional automation in place to pay the invoice following the company’s standard protocols.
2. Improved Machine Maintenance
Because of the heavy usage of automated systems in this approach, it’s very easy for fewer people to operate a larger facility. When a welding robot, as an example, is starting to record readings that indicate it may need a nozzle changed in the upcoming future, it’s able to send a message requesting maintenance to the people operating the business. The assembly line hasn’t stopped yet, it’s just providing a warning that if the maintenance isn’t handled, it will. This allows the people to work around that issue, perhaps stopping the assembly line for an hour to handle all the maintenance requests that have come in that day, rather than facing a staggering start-stop-start-stop process to handle them one at a time as the assembly line machinery fails again and again. At the same time, because maintenance and small repairs are handled before they become a crisis, the machinery lasts longer and is kept in peak condition for longer, because its needs are met before a human operator would typically realize that there was any problem to begin with.
3. Reduced Downtime
When Henry Ford introduced the concept of the assembly line to the world during the second Industrial Revolution, using electricity to keep the vehicles moving along as each worker added their required part, it was a revolutionary idea. Instead of building one vehicle from start to finish in small teams, with a lot of shared experience, his Model A simply required each worker to know what to do with their part. This created significant economy in terms of getting products completed more quickly, but it also introduced the concept of downtime.
If one worker had a problem at their station, the entire factory screamed to a halt. Until that problem was fixed, it couldn’t move again. Under digital transformation and machine learning, human operators monitoring the entire line are informed when a piece of equipment starts to head out of its normal performance, even if it hasn’t gone out of its specifications yet. As with our example above, this allows all the maintenance and repair tasks to be handled in a much shorter, planned window of time, so the manufacturing plant can continue to operate smoothly the rest of the time.
4. Improve Quality Control and Reduce Recalls
Recalls are a pain, yet they still happen frequently. This frustrates customers, hurts your brand and cuts significantly into your profitability. However, machine learning can be combined with a range of other digital transformation technologies to help improve quality control and reduce the number of recalls that you need to perform. As an example, using our industrial robot welders we’ve mentioned above, human operators will receive a notification when a welding robot starts to drift off its normal operating parameters, whether in increased power consumption, increased splatter, poor welds and similar metrics. Although this may not be noted by humans monitoring the machinery, these issues can be caught by the close attention of an analytics program and stopped before the situation decays to the point of causing quality control issues.
Instead of having sent countless products out the door that have borderline welding jobs, which will later result in a recall as the welding jobs fail and cause potential injury to your customers, the problem is caught much earlier in the process and can be corrected before the welding degrades to that point. Because the recall is avoided, you’re reducing waste, damage to your brand’s reputation and expenses at the same time. This approach was developed in the Toyota Manufacturing Technique to help reduce waste in the company and improve profitability. The result is some of the most reliable vehicles on the market.
By understanding how machine learning can make your operation significantly more efficient and cost-effective, you’ll be able to create and implement machine learning in manufacturing in an intelligent fashion as part of your overall digital transformation process. Many of today’s modern machines are already set up with options for industrial IoT and machine learning, but as companies go through the growing pains of digital transformation, they may realize that they’ve purchased the wrong equipment for their long-term needs. Why not take advantage of that situation by looking for the equipment you need on our website? The variety of equipment available on The Equipment Hub provides you with all the options you may want to consider for your business’ digital transformation on a smaller budget.
Sign Up for Our Newsletter
Sign up for our newsletter using the form below to get company insights and updates directly in your inbox!